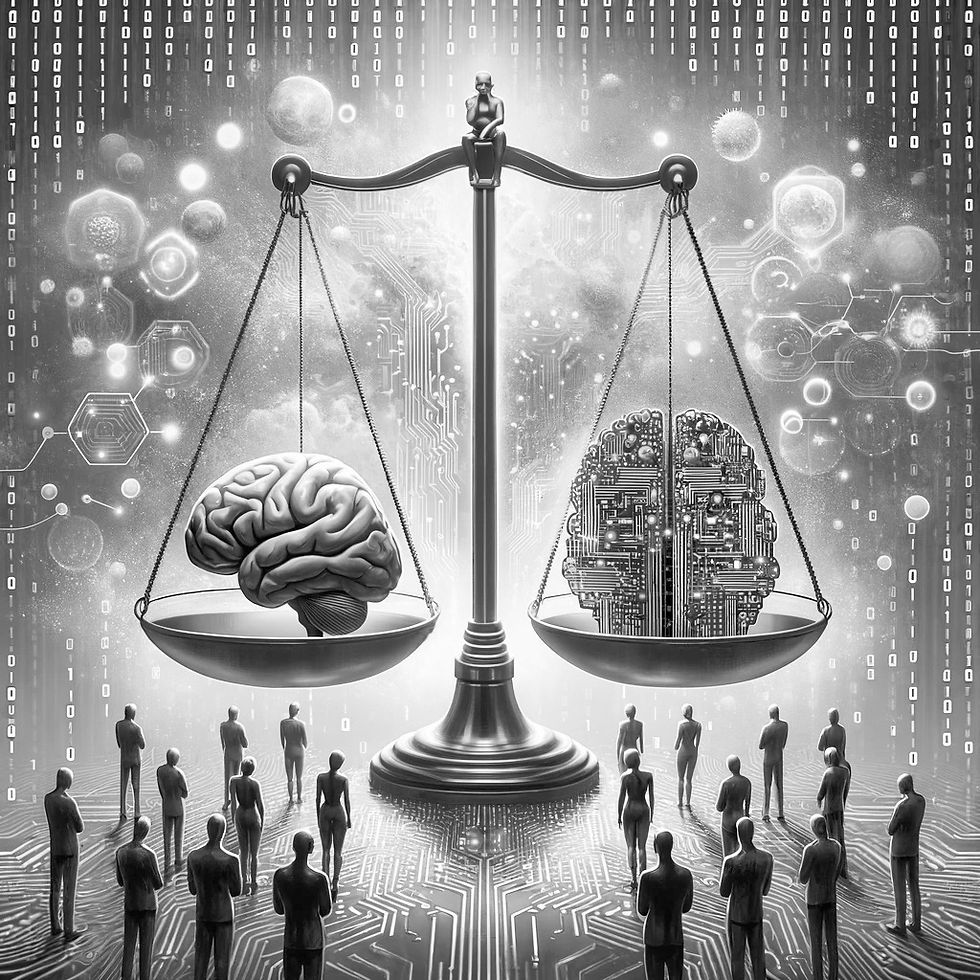
Introduction
The rapid advancement and integration of AI systems in critical sectors—healthcare, finance, law enforcement, and more have sparked intense debates on morality, equity, and the very nature of human autonomy. The promise of AI to deliver unparalleled efficiencies comes with the risk of amplifying societal inequalities, compromising personal privacy, and challenging our concepts of accountability. Algorithms shaping parole, job, and housing decisions often act as mirrors to society's ingrained biases, subtly perpetuating the very disparities they were programmed to transcend. This blog post seeks to navigate the ethical labyrinth that surrounds AI, examining the juxtaposition of its potential to both uplift and undermine society.
What are the ethical dilemmas of artificial intelligence?
1. Bias and Fairness
This report investigates the inherent biases in Artificial Intelligence (AI) systems, highlighting two primary sources. First, the subjective biases of developers can inadvertently be coded into AI algorithms, reflecting personal biases rather than objective analysis. Second, the historical data used to train AI often needs more diversity, failing to represent the full spectrum of human society. Such datasets can perpetuate existing societal biases when used to train AI systems.
Case Study: Analyzing Bias in Text-to-Image Generation Models
The emergence of text-to-image generation technologies, exemplified by models such as OpenAI's DALL-E 2 and Stability AI's Stable Diffusion, represents a significant advancement in artificial intelligence, enabling the generation of images from textual descriptions. Despite the technological breakthroughs, an analysis of these models reveals the existence of embedded biases, particularly concerning gender and racial representations in professional and influential contexts. For instance, queries related to executive roles frequently yield images that disproportionately depict males, illustrating a gender bias. Moreover, the generation of images for "influential person" predominantly features older white males, thereby indicating racial bias. These findings underscore the critical challenge of addressing and mitigating societal biases within AI systems, highlighting the necessity for developing and utilizing diverse and inclusive training datasets. This endeavor is paramount to ensuring that AI-generated content fosters equitable representations and challenges prevailing stereotypes, rather than reinforcing them.
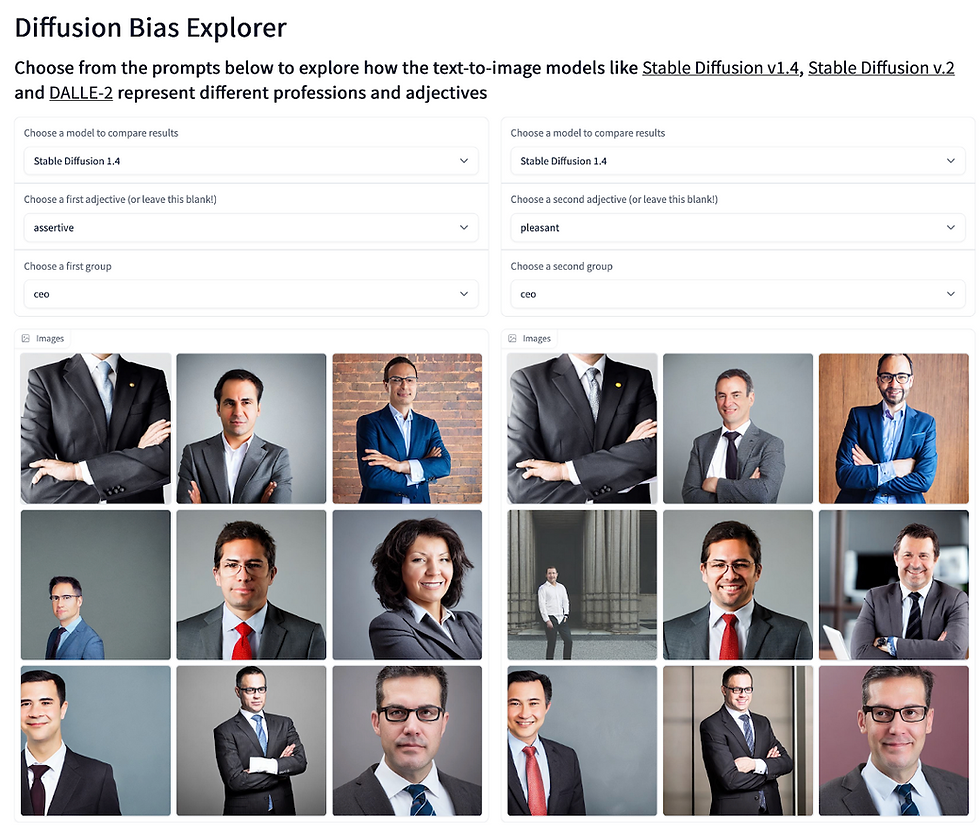
Case Study: Fairness of AI-based Amazon's Recruitment Tool
Amazon's venture into using artificial intelligence for recruitment is a compelling study on the challenges of ensuring fairness in AI applications. Initiated in 2014, the project aimed to automate job application reviews by scoring candidates, but it soon encountered a critical flaw: gender bias. The AI had been trained on a decade's worth of data, predominantly from male applicants, leading it to favor men over women. Attempts were made to neutralize this bias, such as editing the system to ignore gender-related terms. However, these measures failed to address the deep-seated bias fully. By 2015, it was evident that the AI could not reliably perform in a gender-neutral manner, leading to the project's discontinuation. Although recruiters briefly used the tool, it never became a standalone solution for Amazon's hiring process. This episode highlights the intricate challenge of developing unbiased AI systems and the importance of diverse and inclusive training datasets to achieve equitable AI outcomes.
2. Privacy and Surveillance
The introduction of emerging technologies frequently involves complex privacy issues, with artificial intelligence (AI) technology introducing a distinct and unprecedented array of challenges. The concerns surrounding privacy in the context of AI echo those associated with the era of big data and are also significantly amplified. AI distinguishes itself through its advanced capabilities to process extensive datasets, engage in autonomous learning, evolve through adaptive modeling, and deliver actionable insights and predictions. A critical issue, however, is the opacity of these processes. AI makes decisions in ways that are often neither transparent nor easily interpretable, raising intricate questions about the methodologies it employs to analyze and learn from data.
Case Study: Surveillance of Communities of Color/Race
Surveillance practices across the globe, both historical and contemporary, reflect deep-seated issues of racial bias and discrimination. From the monitoring of civil rights activists in the United States to the scrutiny of Black Lives Matter proponents, these actions highlight a broader, international pattern where surveillance disproportionately affects communities of color. Globally, initiatives resembling the U.S.'s China Initiative have led to concerns over profiling and unjust targeting based on nationality, such as the scrutiny of the Chinese diaspora under national security pretexts. These examples underscore the importance of developing surveillance policies that are sensitive to historical injustices and current disparities, ensuring that the application of such technologies is equitable and respects the dignity of all individuals, regardless of their racial or ethnic background.
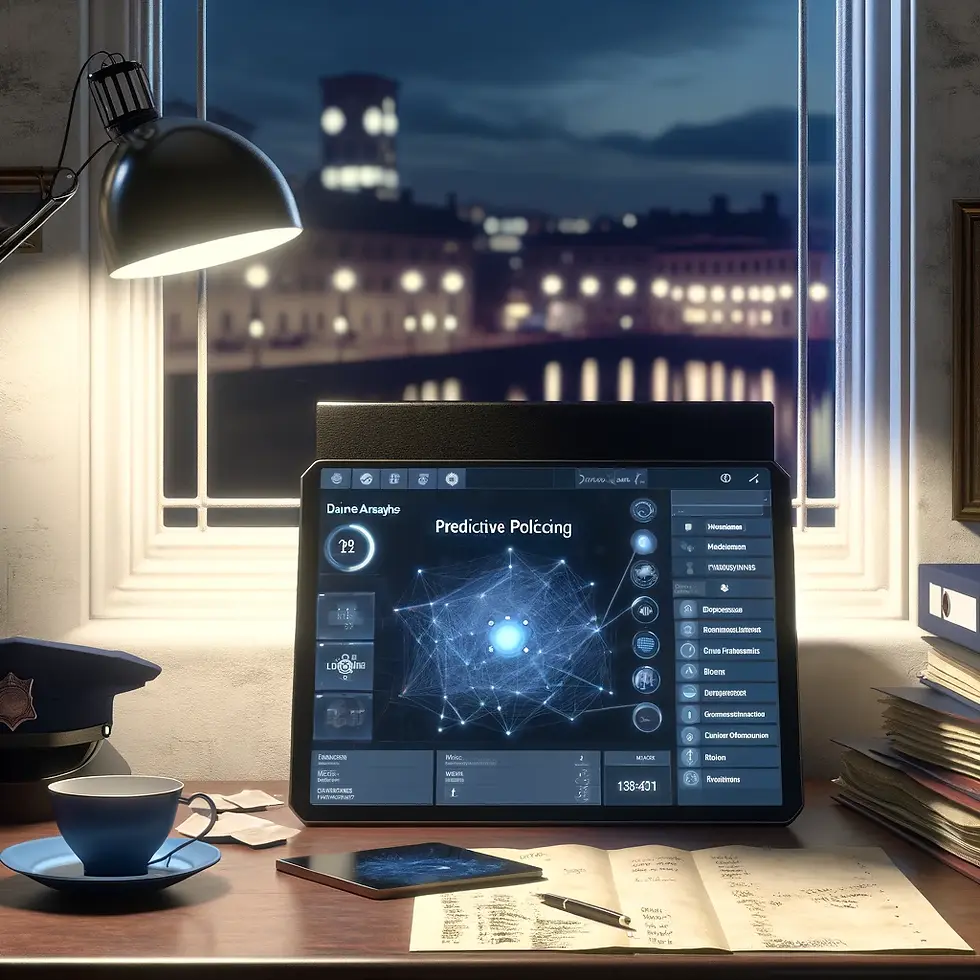
Case Study: AI in Law Enforcement
The deployment of Artificial Intelligence (AI) in areas such as predictive policing and urban surveillance, exemplified by the monitoring of mask usage in Paris's metro, illustrates a complex interplay between bolstering public safety and preserving individual privacy and freedoms. These AI applications offer significant potential to enhance law enforcement effectiveness and promote public health, yet they simultaneously pose risks to privacy and civil liberties. The ethical and privacy concerns surrounding these technologies highlight the urgent need for comprehensive regulations and transparency. Effective governance must ensure these innovations are utilized responsibly, safeguarding against misuse while maintaining public trust and upholding the principles of privacy and freedom.
3. Accountability and Transparency
The opaque nature of many AI systems, often referred to as the "black box" problem, complicates efforts to ensure accountability. When AI systems make decisions with profound impacts on individuals' lives, understanding the rationale behind these decisions is crucial for fairness and trust. In the European Union, the General Data Protection Regulation (GDPR) addresses this directly by granting individuals the right to explanation for decisions made by automated systems. This represents an attempt to legislate against the "black box" nature of AI, requiring systems to be designed in a way that their decisions can be explained and justified.
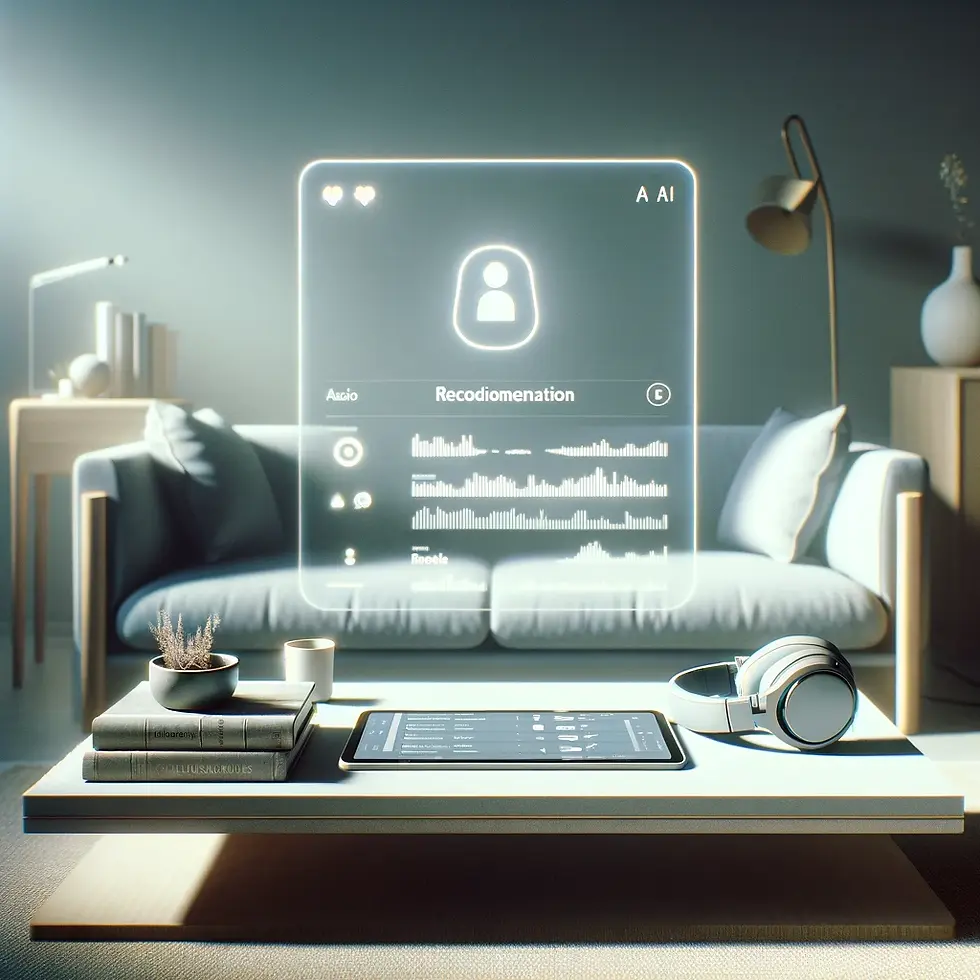
Case Study: AI-Driven Recommender Systems
Recommender systems, integral to digital platforms like Amazon, Netflix, Google, and Facebook, leverage vast personal data, including browsing and viewing habits, to tailor content suggestions. These systems' expansion into smart cars' infotainment technologies raises profound concerns over data transparency, privacy, and security, essentially transforming these innovations into sophisticated tracking mechanisms. Independent audits also play a crucial role in ensuring these systems operate within ethical bounds, safeguarding user privacy while maintaining integrity and trust in AI-driven technologies. This holistic approach underscores the imperative for a balanced integration of AI, where user rights and data ethics are at the forefront of technological advancements.
4. Autonomy and Human Agency
As AI systems take on more tasks, there is concern over the erosion of human decision-making and the autonomy of AI. Balancing the efficiency and capabilities of AI with the need to preserve human control and agency presents a complex ethical challenge. The complex ethical landscape of integrating AI into significant aspects of societal functioning emphasizes the importance of developing AI technologies that are transparent, accountable, and designed to enhance rather than diminish human agency, ensuring that AI serves as a tool for augmenting human capabilities without compromising ethical standards or human autonomy.
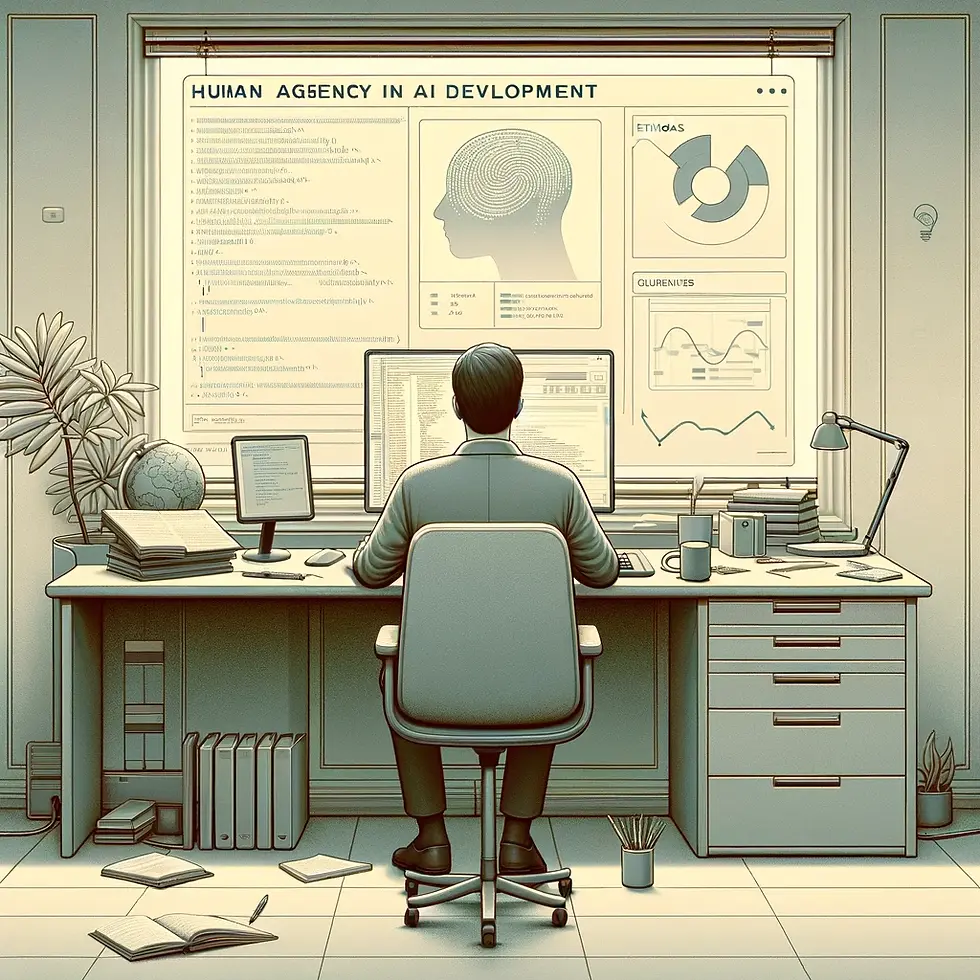
Case Study: COMPAS in the US Criminal Justice System
The use of the COMPAS algorithm for assessing the likelihood of reoffending among individuals in the US criminal justice system. Studies and investigations have revealed that the tool might exhibit racial bias, with implications for sentencing and bail decisions. The inability to fully understand how COMPAS makes its assessments has raised significant concerns over fairness and accountability in its use. The analysis also showed that even when controlling for prior crimes, future recidivism, age, and gender, black defendants were 45 percent more likely to be assigned higher risk scores than white defendants.
Addressing the Dilemmas: Towards Ethical AI
Addressing the ethical dilemmas posed by artificial intelligence (AI) is a complex but essential task, one that requires a coordinated, multi-faceted approach to ensure that AI development aligns with human values and rights. Let's delve deeper into the strategies mentioned:
1. Increasing Interdisciplinary Collaboration
AI's ethical challenges span various dimensions of human life, necessitating input from various disciplines. For instance, ethicists can provide insights into the moral implications of AI decisions, while technologists focus on the feasibility and implementation of ethical AI systems. Legal experts are crucial for understanding and navigating the regulatory landscape, and social scientists can offer perspectives on AI's societal impacts. This collaborative approach ensures that AI technologies are developed and deployed in a manner that is socially responsible, ethically sound, and legally compliant.
2. Developing Robust Ethical Frameworks
Creating ethical guidelines and frameworks for AI involves identifying core values and principles that should guide AI development, such as fairness, accountability, transparency, and respect for human rights. These frameworks serve as a blueprint for developers and stakeholders, helping to anticipate and mitigate ethical risks in AI systems. For example, the IEEE's Global Initiative on Ethics of Autonomous and Intelligent Systems offers a comprehensive set of ethical guidelines aimed at ensuring that AI technologies promote human well-being and minimize harm.
3. Fostering Transparency and Public Engagement
Transparency in AI systems means making the workings of AI algorithms understandable to users and stakeholders, and ensuring that decisions made by AI can be explained and justified. Public engagement, on the other hand, involves dialogues between AI developers, policymakers, and the broader community about the ethical implications of AI. This could take the form of public consultations, forums, and educational campaigns to demystify AI and address public concerns. Engaging with the public ensures that diverse viewpoints are considered and that AI development is aligned with societal values and needs.
4. Implementing Regulatory and Oversight Mechanisms
Regulatory and oversight mechanisms are essential for holding AI systems and their developers accountable. This could involve establishing AI ethics boards within organizations, conducting independent audits of AI systems, and creating legal frameworks that enforce ethical AI practices. For instance, the European Union's General Data Protection Regulation (GDPR) includes provisions for the ethical handling of personal data, indirectly impacting how AI systems process such data. Effective oversight mechanisms ensure compliance with ethical standards and provide remedies for addressing violations.
Legislative Interest in AI
The legislative landscape around AI is evolving, with significant increases in AI-related bills passed and mentions of AI in legislative proceedings worldwide. This trend reflects a growing recognition of the importance of AI governance.
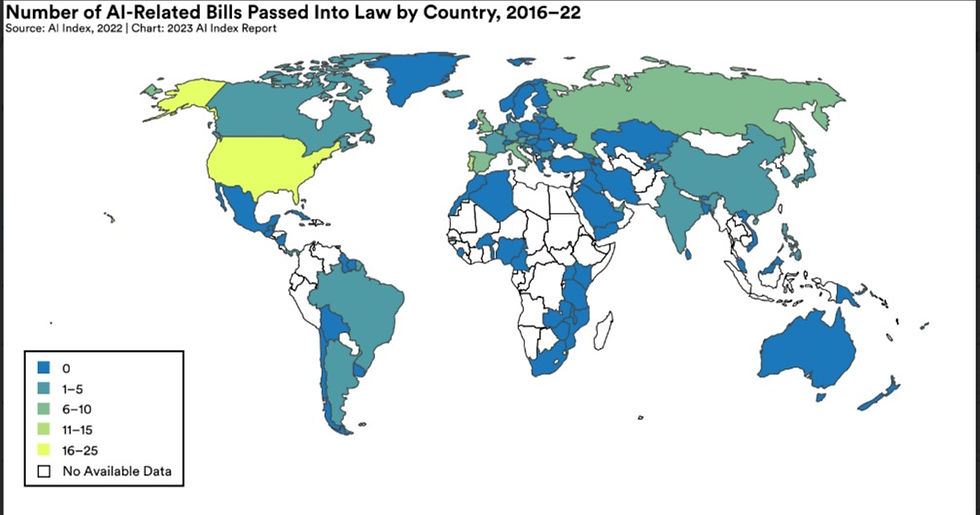
The table contains information on AI-related legislation from select countries in 2022 from the Stanford AI Index Report 2023.
Bill Name | Description | |
Kyrgyz Republic | About the Creative Industries Park | This law determines the legal status, management, and operation procedures of the Creative Industries Park, established to accelerate the development of creative industries, including artificial intelligence. |
Latvia | Amendments to the National Security Law | A provision of this act establishes restrictions on commercial companies, associations, and foundations important for national security, including a commercial company that develops artificial intelligence. |
Philippines | Second Congressional Commission on Education (EDCOM I) Act | A provision of this act creates a congressional commission to review, assess, and evaluate the state of Philippine education; recommend innovative and targeted policy reforms in education; and to appropriate funds. The act calls for reforms to meet the new challenges to education caused by the Fourth Industrial Revolution characterized, in part, by the rapid development of artificial intelligence. |
Spain | Right to Equal Treatment and Non-discrimination | A provision of this act establishes that artificial intelligence algorithms involved in public administrations’ decision-making take into account bias-minimization criteria, transparency, and accountability, whenever technically feasible. |
United States | AI Training Act | This bill requires the Office of Management and Budget to establish or otherwise provide an AI training program for the acquisition workforce of executive agencies (e.g., those responsible for program management or logistics), with exceptions. The purpose of the program is to ensure that the workforce knows the capabilities and risks associated with AI. |
Please note that the descriptions provided in the table are summaries of the legislation and not the full text.
Conclusion
The journey towards ethical AI is ongoing and requires concerted efforts from all sectors of society. By fostering interdisciplinary collaboration, developing robust ethical frameworks, enhancing transparency, engaging the public, and implementing stringent regulatory measures, we can navigate the ethical challenges posed by AI. This balanced approach ensures that AI technologies advance in a way that respects human dignity, promotes societal welfare, and upholds the principles of justice and equity, paving the way for a future where AI serves the greater good.
Comments